Suppose we observe a training set
{Dox-(1,ail,rar . . , rip)) for i-l, m ,n subjects. We want to fit a linear
regression model /(x) otXI ,, to the training data to minimize the residual sum
ofsquares RSS(β) Σǐ 1 (Vi r β)2 where β = (A 屆,-. W pm (a)(10%) Please
derive the solution ßt. arg ming RSS(β) in terms of Y-|h li) Ar (b)(10%) in
ridge regression, we find ßridge rgminß RSS(β) subject to Σ./ Please write out
the equivalent Lagrangian form of the optimization problem. st. (c) (10%)
Please derive the form of ßridge in terms of Y # (yī and the Lagrange
multiplier λ YnF, X x(zh t
ANSWER
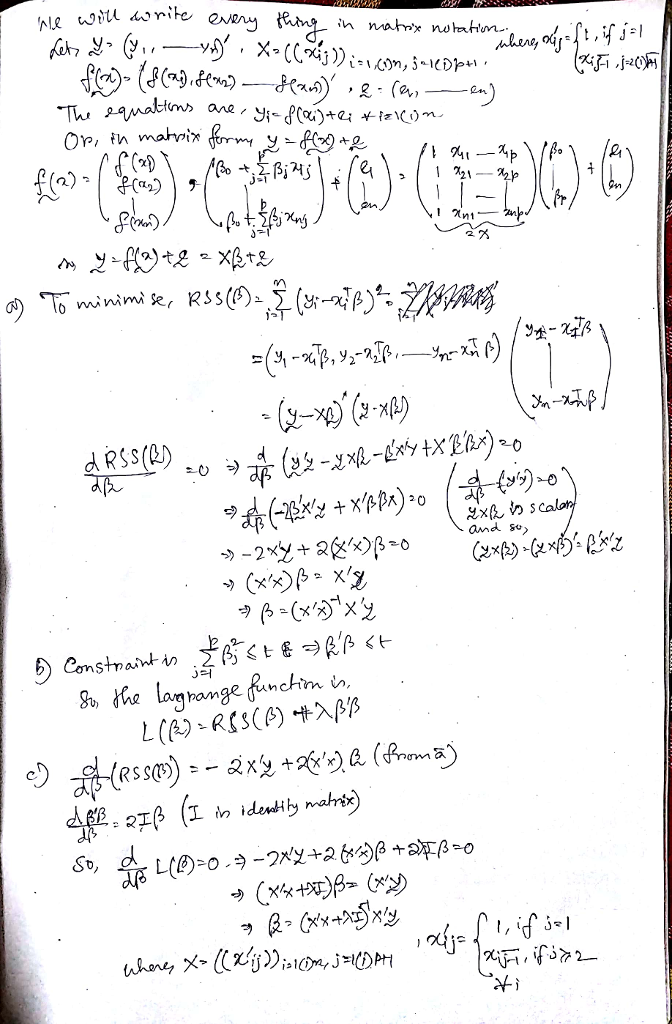
Comments
Post a Comment